Gokul Ravi and coauthors present new advances in quantum computing, win Best Paper Award at IEEE Quantum Week
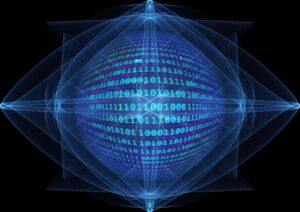
Gokul Subramanian Ravi, assistant professor of computer science and engineering at the University of Michigan, and his coauthors are presenting innovative new research on quantum computing at the 2023 IEEE International Conference on Quantum Computing and Engineering (QCE), otherwise known as IEEE Quantum Week. Prof. Ravi’s three papers at the conference cover the extension of zero-noise extrapolation to fault-tolerant quantum computing, the development of a new runtime technique for variational quantum algorithms, and the application of permutation symmetry to variational quantum circuits.
IEEE Quantum Week, taking place September 17-22 in Bellevue, WA, is the premier international venue for multidisciplinary quantum computing experts to come together and share their latest findings and developments. Aiming to bridge the gap between scientific study and technological development, the conference unites researchers, engineers, entrepreneurs, developers, and others from across academia and industry to foster collaboration and advance the field.
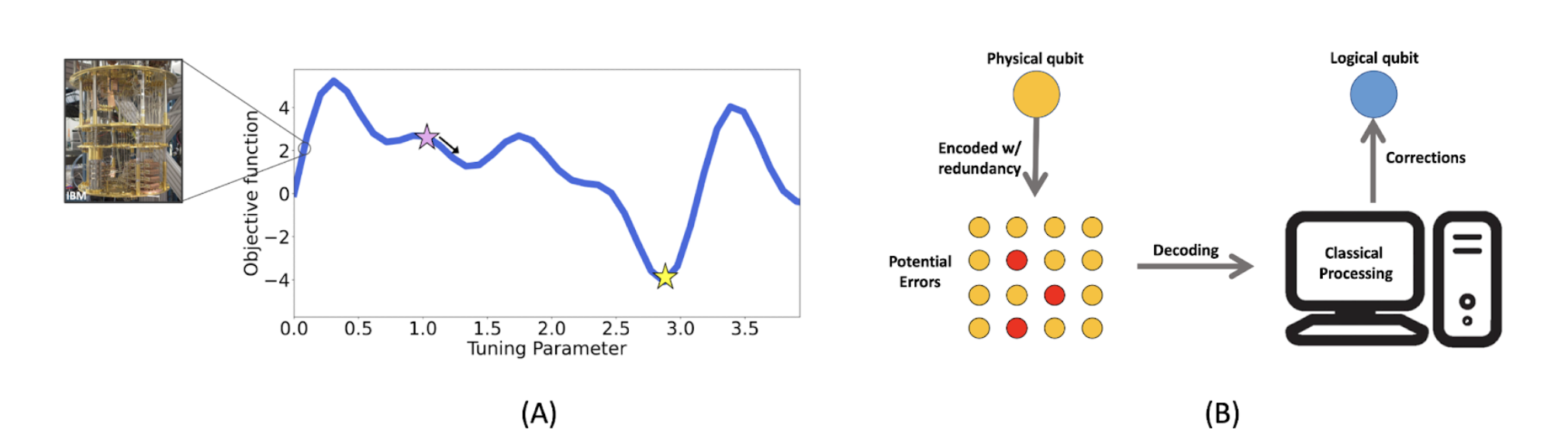
Prof. Ravi, who joined the CSE faculty this fall and is heading the Computer Architecture For Quantum Advantage (CAFQA) Lab, has a strong record of groundbreaking research in quantum computing, with a particular focus on quantum computing architecture at the intersection of quantum and classical computing systems. His three new papers at IEEE Quantum Week yield even further advances in these areas.
Zero noise extrapolation on logical qubits by scaling the error correction code distance
Misty A. Wahl, Andrea Mari, Nathan Shammah, William J. Zeng, Gokul Subramanian Ravi
Abstract: In this work, we migrate the quantum error mitigation technique of Zero-Noise Extrapolation (ZNE) to fault-tolerant quantum computing. We employ ZNE on logically encoded qubits rather than physical qubits. This approach will be useful in a regime where quantum error correction (QEC) is implementable but the number of qubits available for QEC is limited. Apart from illustrating the utility of a traditional ZNE approach (circuit-level unitary folding) for the QEC regime, we propose a novel noise scaling ZNE method specifically tailored to QEC: distance scaled ZNE (DS-ZNE). DS-ZNE scales the distance of the error correction code, and thereby the resulting logical error rate, and utilizes this code distance as the scaling `knob’ for ZNE. Logical qubit error rates are scaled until the maximum achievable code distance for a fixed number of physical qubits, and lower error rates (i.e., effectively higher code distances) are achieved via extrapolation techniques migrated from traditional ZNE. Furthermore, to maximize physical qubit utilization over the ZNE experiments, logical executions at code distances lower than the maximum allowed by the physical qubits on the quantum device are parallelized to optimize device utilization. We validate our proposal with numerical simulation and confirm that ZNE lowers the logical error rates and increases the effective code distance beyond the physical capability of the quantum device. For instance, at a physical code distance of 11, the DS-ZNE effective code distance is 17, and at a physical code distance of 13, the DS-ZNE effective code distance is 21. When the proposed technique is compared against unitary folding ZNE under the constraint of a fixed number of executions of the quantum device, DS-ZNE outperforms unitary folding by up to 92% in terms of the post-ZNE logical error rate.
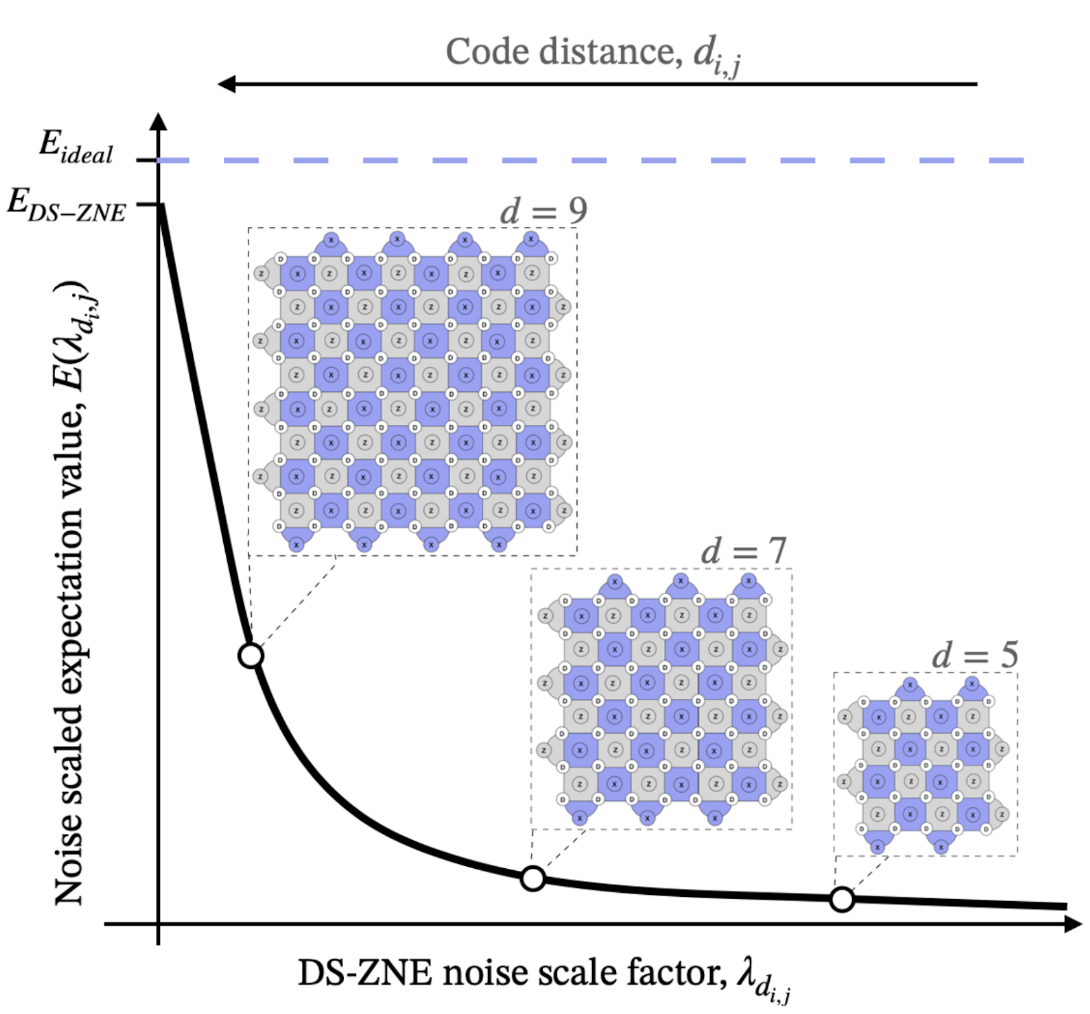
DISQ: Dynamic Iteration Skipping for Variational Quantum Algorithms
Junyao Zhang, Hanrui Wang, Gokul Subramanian Ravi, Frederic T. Chong, Song Han, Frank Mueller, Yiran Chen
Abstract: This paper proposes DISQ to craft a stable landscape for VQA training and tackle the noise drift challenge. DISQ adopts a “drift detector” with a reference circuit to identify and skip iterations that are severely affected by noise drift errors. Specifically, the circuits from the previous training iteration are re-executed as a reference circuit in the current iteration to estimate noise drift impacts. The iteration is deemed compromised by noise drift errors and thus skipped if noise drift flips the direction of the ideal optimization gradient. To enhance noise drift detection reliability, we further propose to leverage multiple reference circuits from previous iterations to provide a well founded judge of current noise drift. Nevertheless, multiple reference circuits also introduce considerable execution overhead. To mitigate extra overhead, we propose Pauli-term subsetting (prime and minor subsets) to execute only observable circuits with large coefficient magnitudes (prime subset) during drift detection. Only this minor subset is executed when the current iteration is drift-free. Evaluations across various applications and QPUs demonstrate that DISQ can mitigate a significant portion of the noise drift impact on VQAs and achieve 1.51-2.24x fidelity improvement over the traditional baseline. DISQ’s benefit is 1.1-1.9x over the best alternative approach while boosting average noise detection speed by 2.07x.
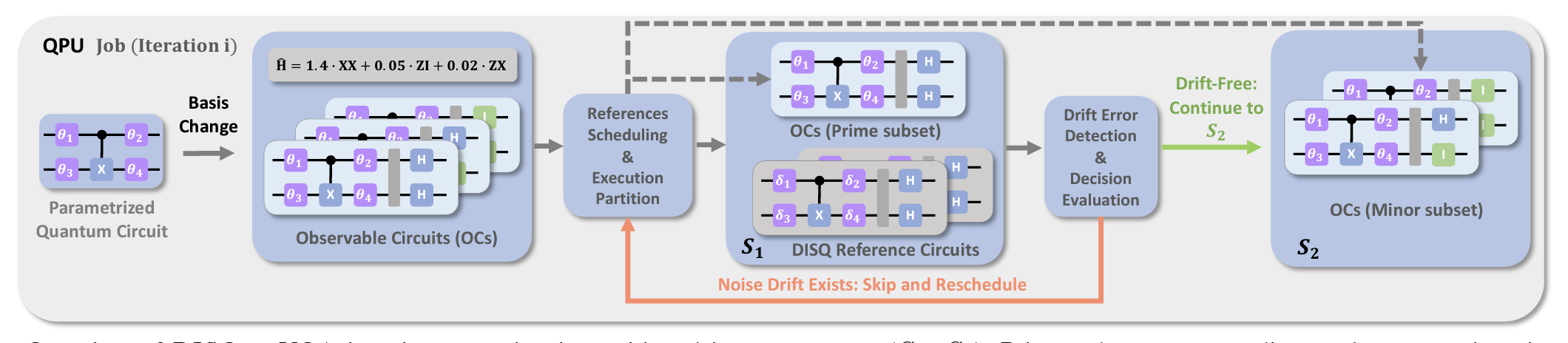
Benchmarking variational quantum circuits with permutation symmetry
Han Zheng, Christoper Kang, Gokul Subramanian Ravi, Hanrui Wang, Kanav Setia, Frederic T. Chong, Junyu Liu
This paper was honored with a Best Paper Award in the Quantum Algorithms track at the conference.
Abstract: We propose SnCQA, a set of hardware-efficient variational circuits of equivariant quantum convolutional circuits respective to permutation symmetries and spatial lattice symmetries with the number of qubits n. By exploiting permutation symmetries of the system, such as lattice Hamiltonians common to many quantum many-body and quantum chemistry problems, Our quantum neural networks are suitable for solving machine learning problems where permutation symmetries are present, which could lead to significant savings of computational costs. Aside from its theoretical novelty, we find our simulations perform well in practical instances of learning ground states in quantum computational chemistry, where we could achieve comparable performances to traditional methods with few tens of parameters. Compared to other traditional variational quantum circuits, such as the pure hardware-efficient ansatz (pHEA), we show that SnCQA is more scalable, accurate, and noise resilient (with 20× better performance on 3×4 square lattice and 200%−1000% resource savings in various lattice sizes and key criterions such as the number of layers, parameters, and times to converge in our cases), suggesting a potentially favorable experiment on near-time quantum devices.